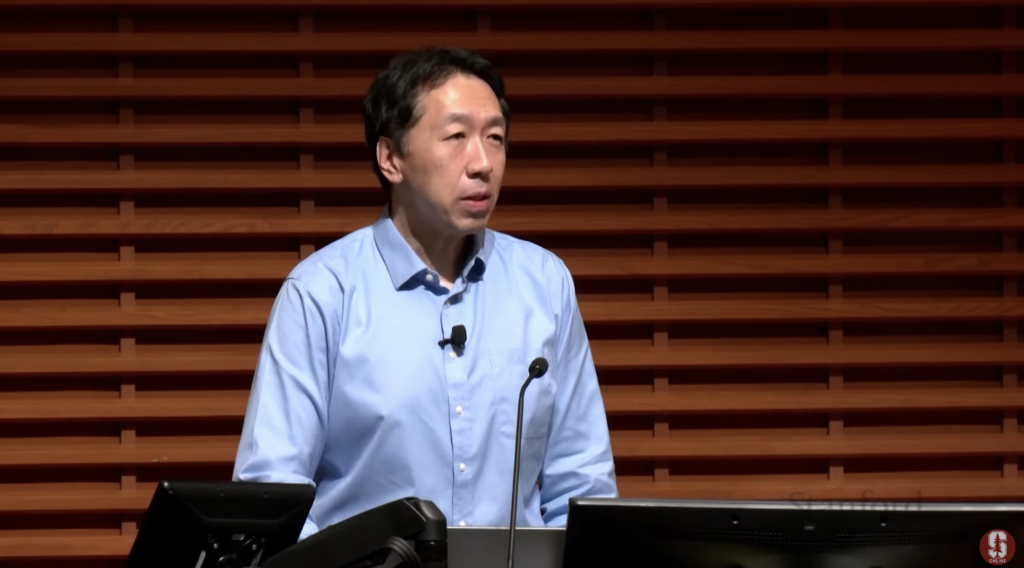
Andrew Ng: Opportunities in AI - 2023
Stanford Online
https://www.coursera.org/specializations/machine-learning-introduction
This discussion took place on July 26, 2023, at Cemex Auditorium, Stanford University, and was hosted by the Stanford Graduate School of Business.
This talk covers:
- Trends in AI Technologies and Tools
- Supervised Learning
- Generative AI
- The Adoption of AI
- Opportunities in AI
- Process for Building Startups
- AI Risks and Social Impact
https://www.coursera.org/specializations/machine-learning-introduction
Summarized by ChatGPT:
Dr. Andrew Ng, a prominent figure in AI, was introduced as a managing general partner of AI Fund, founder of DeepLearning.AI and Landing AI, chairman and co-founder of Coursera, and an adjunct professor of Computer Science at Stanford. He previously led the Google Brain team and was the director of the Stanford AI lab. His influence on AI education has impacted millions of people worldwide.
In his talk, Dr. Ng discussed AI as a general-purpose technology, akin to electricity, with applications spanning various domains. He emphasized that AI comprises a collection of tools, with a focus on supervised learning and generative AI as the most important ones. Supervised learning excels at recognizing and labeling things, while generative AI is an exciting recent development. These tools offer numerous opportunities in the AI landscape.
Supervised learning involves creating mappings from inputs to outputs, such as labeling emails as spam or not spam, predicting user interactions with online ads, or identifying defects in manufactured products. It has a wide range of applications, including self-driving cars, ship route optimization, and sentiment analysis of restaurant reviews.
The typical workflow for a supervised learning project includes collecting labeled data, training an AI model, and deploying it to make predictions. In recent years, the focus has shifted towards large-scale supervised learning, where using powerful hardware and vast datasets has led to significant improvements in AI model performance.
The current decade is characterized by combining supervised learning with generative AI, allowing systems like ChatGPT to generate text based on input prompts. Generative AI relies on supervised learning to predict the next word or subword, enabling the creation of large language models. This approach has driven remarkable progress in the field of AI.
The speaker discusses the significance of large language models, emphasizing their role not only as consumer tools but also as developer tools. They explain that these models have the potential to significantly accelerate the development of AI applications, reducing the timeline from months to mere minutes or hours. The speaker provides an example of building a sentiment classifier using a prompt-based approach, highlighting how it can be accomplished quickly and easily. They also discuss the growing importance of generative AI and its potential for creating valuable applications. The speaker concludes by emphasizing the long-term value creation opportunities that arise from leveraging generative AI and other advanced AI tools.
The context discusses two key trends related to AI and its adoption. The first trend highlights AI as a general-purpose technology with diverse use cases. It emphasizes that while AI has made significant progress, much of its value is still concentrated in consumer software and the internet, and there’s room for expansion into other industries.
The second trend addresses the challenge of AI adoption outside of tech and consumer software. It points out that the successful recipe for AI projects, which involves hiring a large team of engineers to work on multi-billion dollar projects, doesn’t apply to many industries that lack massive user bases. The context mentions examples like pizza makers and agriculture companies with smaller projects that are economically challenging to implement using the traditional approach.
The exciting aspect of the second trend is the development of low-code and no-code tools that enable end-users to customize AI systems for their specific needs. These tools aim to democratize AI deployment, allowing organizations in various industries to benefit from AI without the need for extensive engineering resources. The context also mentions technologies like text prompting, visual prompting, and data-centric AI as part of this trend.
The speaker highlights the importance of pushing the value of AI beyond the tech world and into all industries, emphasizing that the rest of the economy represents a significant opportunity.
Additionally, the context briefly touches upon the AI stack, with layers including hardware, infrastructure, developer tools, and applications. It suggests that while there is competition in the lower layers, the application layer may offer substantial opportunities with relatively less competition, especially for AI projects that require expertise in both AI and specific domains, such as relationships.
Overall, the context discusses the potential for AI to become a widespread technology, the challenges in adopting AI across different industries, and the role of new tools and approaches in facilitating this expansion.
The speaker discusses the process of building startups and shares a recipe for their development. They emphasize the importance of having a concrete idea to work on and partnering with subject matter experts. They also mention the need to address ethical concerns in AI, particularly regarding job disruption, and downplay the hype around artificial general intelligence (AGI) and AI-related extinction risks. The speaker’s approach is to focus on specific AI applications that benefit humanity.
Translated by ChatGPT:
德安德鲁·吴博士,人工智能领域的杰出人物,被介绍为AI Fund的管理普通合伙人,DeepLearning.AI和Landing AI的创始人,Coursera的主席兼创始人,以及斯坦福大学计算机科学的兼职教授。他曾领导Google Brain团队,并担任斯坦福人工智能实验室的主任。他在人工智能教育方面的影响影响了全球数百万人。
在他的演讲中,吴博士将人工智能比作一种通用技术,类似于电力,可以应用于各个领域。他强调,人工智能由一系列工具组成,其中监督学习和生成式人工智能是最重要的工具。监督学习擅长识别和标记事物,而生成式人工智能是最近的一项令人兴奋的发展。这些工具在人工智能领域提供了许多机会。
监督学习涉及创建从输入到输出的映射,例如将电子邮件标记为垃圾邮件或非垃圾邮件,预测用户与在线广告的互动,或识别制造产品的缺陷。它具有广泛的应用,包括自动驾驶汽车、船舶航线优化和餐厅评论的情感分析。
监督学习项目的典型工作流程包括收集带有标签的数据、训练人工智能模型和部署它以进行预测。近年来,焦点已转向大规模监督学习,在这个领域,使用强大的硬件和大规模数据集已经显著改善了人工智能模型的性能。
当前的十年被描述为将监督学习与生成式人工智能相结合的十年,这使得像ChatGPT这样的系统可以根据输入提示生成文本。生成式人工智能依赖于监督学习来预测下一个单词或子单词,从而实现大型语言模型的创建。这种方法推动了人工智能领域的显著进展。
演讲者讨论了大型语言模型的重要性,强调它们不仅是消费者工具,也是开发者工具。他们解释说,这些模型有潜力显著加速人工智能应用的开发,将时间线从几个月缩短到几分钟或几小时。演讲者提供了使用基于提示的方法构建情感分类器的示例,突出了如何快速轻松地实现这一目标。他们还讨论了生成式人工智能的不断增长重要性,以及其为创建有价值的应用程序提供的潜力。演讲者最后强调了利用生成式人工智能和其他先进人工智能工具产生长期价值创造机会的重要性。
这个上下文讨论了与人工智能及其采用相关的两个关键趋势。第一个趋势强调了人工智能作为一种通用技术,具有各种用途。它强调,虽然人工智能取得了显著进展,但其价值仍然集中在消费者软件和互联网上,还有扩展到其他行业的空间。
第二个趋势解决了在技术和消费者软件以外领域采用人工智能的挑战。它指出,成功的人工智能项目配方,涉及雇佣大量工程师来从事数十亿美元的项目,不适用于许多没有庞大用户群的行业。上下文提到了比萨制造商和农业公司等示例,这些示例有较小的项目,使用传统方法在经济上具有挑战性。
第二个趋势的令人兴奋之处在于开发了低代码和无代码工具,使最终用户能够为其特定需求定制人工智能系统。这些工具旨在使人工智能部署民主化,允许各个行业的组织在不需要大量工程资源的情况下受益于人工智能。上下文还提到了文本提示、视觉提示和数据中心人工智能等技术作为这一趋势的一部分。
演讲者强调了将人工智能的价值推动到技术世界以外和所有行业的重要性,强调了其余的经济体代表了巨大的机会。
此外,上下文简要涉及了人工智能堆栈,包括硬件、基础设施、开发者工具和应用程序等层次。它建议,虽然在较低层次存在竞争,但应用层可能提供了相对较少竞争的重大机会,特别是对于需要在人工智能和特定领域方面拥有专业知识的人工智能项目,比如关系。 总的来说,这个上下文讨论了人工智能成为一种广泛应用技术的潜力,跨足不同行业采用人工智能所面临的挑战,以及新工具和方法在促进这种扩展中的作用。
演讲者讨论了建立初创企业的过程,并分享了他们的发展秘诀。他们强调了拥有具体的创意点子的重要性,以及与领域专家合作的重要性。他们还提到了解决与人工智能相关的伦理问题的必要性,特别是关于工作岗位被替代的问题,并淡化了围绕人工通用智能(AGI)和与人工智能相关的灭绝风险的炒作。演讲者的方法是专注于有益于人类的具体人工智能应用。